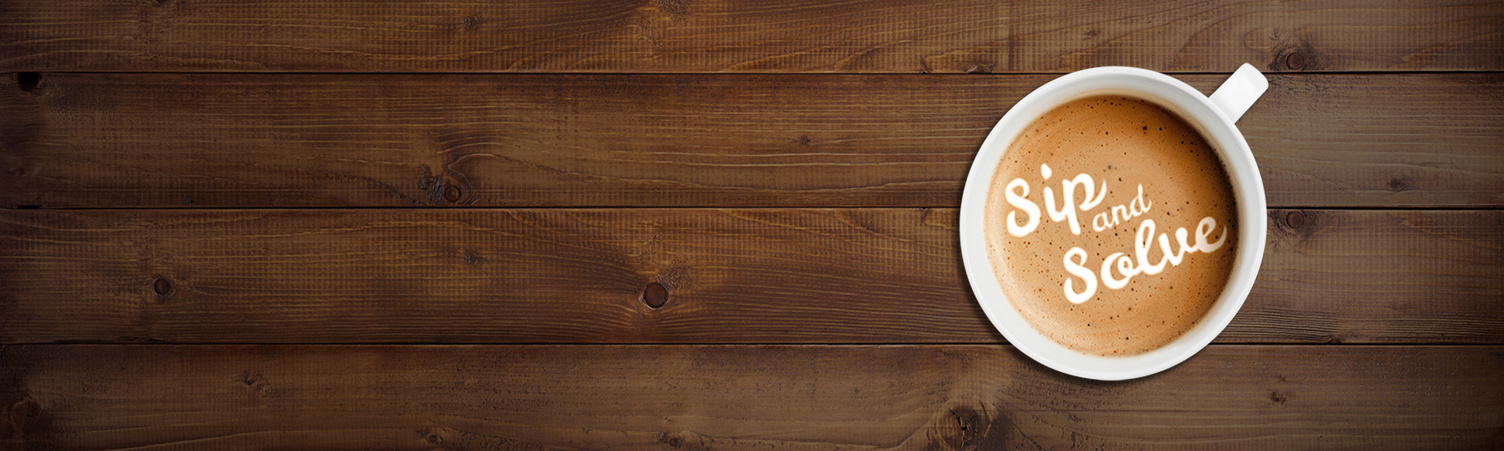
Sip and Solve™
Solving business challenges in a coffee break
Have you ever wondered whether you should consider using a blended scorecard in your small business decisioning?
In this Sip and Solve session I provide an overview of blended decision making, why it’s important and what data can be leveraged to start or enhance your blended data analysis.
Let me start with a little anecdote that I heard recently. A lender had a business apply for a large business loan. The lender checked all the information about the business and reviewed the commercial credit data. Everything fit within their buy box, and so they approved the loan.
Unfortunately, they did not check the consumer credit for the owner of the business, where they might have found some troubling indications of higher risk. The loan went bad, and the lender had to take a 400, 000 loss. Looking at both business and consumer blended credit and financial data when making decisions for small businesses can provide substantial lift over consumer only or commercial only models.
When we think about businesses in the U. S., we tend to think about the large, iconic brands. The Apples and Googles and General Motors types of companies. The truth is that most businesses in the U. S. are small, but they are important because they employ nearly half of U. S. workers. As you can see from this information I pulled from digital. com, there are thirty million small businesses in the U. S., and they have a major impact on the U. S. economy. To grow and prosper, they need access to capital, and lenders need strong methods for assessing risk differently than they would for large corporations.
Let's think about a hypothetical small business as it goes through its life cycle. We have Jane who starts a business with a partner using their own savings. At this earliest stage, using consumer only credit makes the most sense, and is the only data available. The business grows and they get some loans from their families to help them, but at some point, they need to have access to more capital so they can continue growing and expanding, even hiring a worker or two.
For lenders, early on, this business has little history and no credit profile, so they have to rely just upon the consumer credit data, but as the business continues to grow and establish itself, Jane and her partner will rely less on the personal financing and more on financing for the business itself. This is where lenders should use a blended model to properly assess the risk, because it is difficult to know where on this stepping stone here that you are, and when the transitions happen, blended models can really help cover all the bases, even if you don't know exactly where the business fits.
I will note that when looking at business credit and consumer credit when considering small business risk, many of the risk profiles will align. Both business and consumer profiles will have either strong or weak credit. However, an important minority of applicants will have very different consumer and business credit risk profiles, and this is where the value of considering both can generate significant lift in your decision making.
These graphs demonstrate exactly this scenario. On the top graph, I've pulled only business with high credit scores reflecting low risk. As you can see, the left tail of this data shows that some percentage of these businesses have consumers with very low credit scores. You can see 475, 500, very low scores.
Similarly, on the bottom, I've pulled in consumers that have very high credit scores, but their businesses have very low scores, indicating high risk. These are the cases where blended scorecards really have the most value.
So, what are some reasons you might not be using a blended scorecard currently? One of the things that I've heard from lenders is, we don't need one because we manually underwrite all our loans. A blended scorecard even helps in this case because it provides an objective score with a recommendation based on credit policy, which can improve consistency in decision making.
It also helps identify those easy decisions for automated decisioning. Some credit decisions really are easy. The credit risk is low for both business and consumer, so you just approve. Or the credit risk is too high. There are bankruptcies, there are collections, judgments. It's an easy decision to decline and no further review is necessary.
This really frees up underwriter’s time for those more complex cases where their expertise and research are so vital. Or you might also think, we don't have the right data to develop a blended scorecard. This is where Experian can really help. We can match and append archived data to your applications and customers so you can do your own analysis and scorecard development.
Our analysts and data scientists can collaborate with you to build out custom models. And finally, our Ascend Analytical Sandbox brings together years of archived data plus your application and performance data all in one place with necessary analytical tools and packages.
This represents a very simplified process flow for how the decision process works. An application is received by the lender and credit checks initiated. For the business entity and the consumer or consumers who are guarantors for the loan. If a business credit file is found, then data is pulled from it, plus the consumer credit profile and their scores in a blended scorecard.
If there is no hit on the business, like in the example when Jane and her partner first started out, it's not a problem. The application goes through a consumer only scorecard. In either case, the application is then decisioned automatically or is routed to underwriting with further review, and in this case, with a score and with a recommendation based on credit policy.
That’s all great, right? But what types of data should we focus on? There is some information that must come directly from the application because it is either required to match to the credit bureau data. Or because it's valuable information that isn't collected by credit bureaus. So, there are going to be things like name, address, social security number, and or tax IDs for matching.
And things like household income and business revenue that credit bureaus won't have. There's also going to be some items where you're going to take information from the application and combine it with Bureau information, such as consumer debt to income ratios. From the Consumer and Business Bureau data, lenders will often look for information such as credit risk scores, industry, number of inquiries, derogatory credit, delinquent trades, months in business.
Each lender and portfolio will have to analyze the pertinent data and weighting of factors to assess their risk for decisions and pricing purposes. So variable selection in this case is especially important for assessing your own risk.
So, what does all this look like? As you can see here, I've lined up a random sample of some blended business and owner guaranteed data. It includes business and consumer scores, plus some additional potential risk criteria from the business and consumer profiles. This is where we can start to look and see where we have information that matches and where it doesn't necessarily match.
In this view of the same data, I've highlighted some cases in green where the business scores and consumer scores are high and some cases where they're both low. So, these are those easy cases where the decisions are going to be simple to make. It's the cases where they don't match that we want to spend a little bit more time.
Shown here are three examples where we see that mismatch in scores. Again, this is going to be the minority of cases, but they're where you'll find the most lift using this approach. In the first case, the business score is good, but the consumer score is low. Why might that be? We can get further clues about the risk of the business by looking at other information.
On the business side, you can see we have a high balance to limit ratio that we might want to consider as a sign of higher risk. And on the consumer side, we see several recent inquiries indicating that they are seeking additional credit, which could also be a sign of risk. In a blended model, we could consider all these factors with our weighting of data so that we have an actionable and consistent method of making decisions within our risk tolerance.
Sometimes, like in the second case shown here, where we have an extremely low business score and a very high consumer score, the reason for the discrepancy might not be as apparent. This is where the variable selection in the model is so important to ensuring we pick up any relevant data that can be used to make our decision.
We've talked a lot about using blended data to make an initial credit decision, and I might have mentioned once or twice that you might also consider it in initial pricing. But is that it? As you might have guessed, the answer is no. There are opportunities within portfolio management and even in acquisitions.
So, some portfolio management uses for blended data are making renewal decisions, proactive credit line increases and decreases, identifying cross sell opportunities, and even identifying early warning signs within your portfolio. I'll give another quick real-world example. I was working on a cross sell targeting model, and I wanted a population with a very high probability of approval. My target rate was 90%. So based on my analysis, my prediction was that the population I'd found would have an approval rate of closer to 92 to 93 percent. I suspected that it would be a little bit lower because of adverse selection, but I wasn't expecting my approval rate would end up being only 78 to 80 percent at the end of the campaign.
So, what was the problem? I started digging through the application decisions and I realized when looking at the data That, although we used a blended scorecard to make the decision, when I came up with my targeting model, I had only used business data, and I therefore missed a lot of information that could have helped me have a better, more targeted campaign to those people that I wanted to make sure we're getting approved.
This is all great. Now what do we do next steps include answering the following questions for yourself? Where are you in the process right now? And do you have any gaps? And that might be in the analytics, or it might be in the data. Do you currently have the right analytics, or could you benefit from some assistance from someone like Experian?
What might you need from a data and infrastructure perspective? Are you currently collecting the right information on your application? So, as we discussed, this would be things like non bureau data for the scorecard, like income, business revenue, et cetera. Also, information required for Bureau matching.
So, on the business side, we require a name, tax ID, and address to match. And on the consumer or consumers, we need the name, address, Social Security number, birth date, and permission for running the credit.
I want to thank you for spending your coffee break with me today. I really hope this was useful information. And that you're able to walk away with at least a few ideas about how this does or could work within your organization.
Emily Garrett provides strategic analytical consulting for the Ascend Analytical Sandbox in the Commercial Data Science team at Experian. She is focused on providing customized analytic support to clients to drive growth in their portfolios. Prior to joining Experian, Emily worked as a senior level analyst responsible for small business credit card acquisitions and origination strategy for PNC Bank.
We will be scheduling more Sip and Solve sessions. Sign up below to be notified.
This site is protected by reCAPTCHA and the Google Privacy Policy and Terms of Service apply.