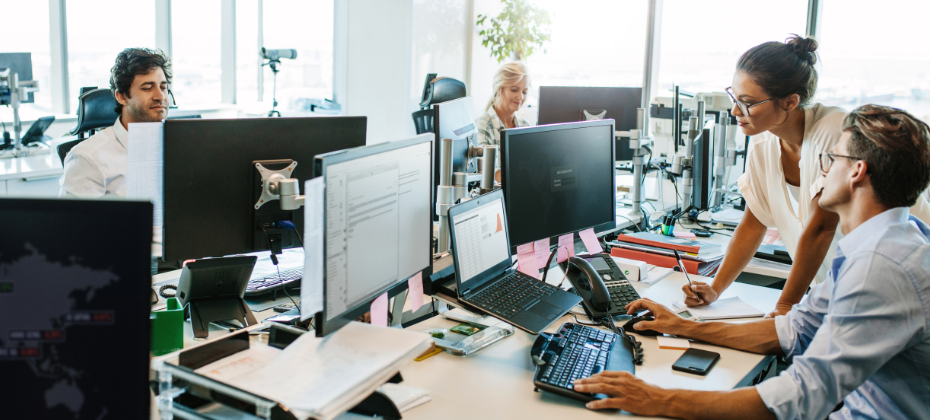
Using data to understand risk and make lending decisions has long been a forte of leading financial institutions. Now, with artificial intelligence (AI) taking the world by storm, lenders are finding innovative ways to improve their analytical capabilities.
How AI analytics differs from traditional analytics
Data analytics is analyzing data to find patterns, relationships and other insights.
There are four main types of data analytics: descriptive, diagnostic, predictive and prescriptive. In short, understanding the past and why something happened, predicting future outcomes and offering suggestions based on likely outcomes.
Traditionally, data analysts and scientists build models and help create decisioning strategies to align with business needs. They may form a hypothesis, find and organize relevant data and then run analytics models to test their hypothesis.
However, time and resource constraints can limit the traditional analytics approach. As a result, there might be a focus on answering a few specific questions: Will this customer pay their bills on time? How did [X] perform last quarter? What are the chances of [Y] happening next year?
AI analytics isn’t completely different — think of it as a complementary improvement rather than a replacement. It relies on advances in computing power, analytics techniques and different types of training to create models more efficient than traditional analytics.
By leveraging AI, companies can automate much of the data gathering, cleaning and analysis, saving them time and money. The AI models can also answer more complex questions and work at a scale that traditional analytics can’t keep up with.
Advances in AI are additionally offering new ways to use and interact with data. Organizations are already experimenting with using natural language processing and generative AI models. These can help even the most non-technical employees and customers to interact with vast amounts of data using intuitive and conversational interfaces.
Benefits of AI analytics
The primary benefits of AI-driven analytics solutions are speed, scale and the ability to identify more complex relationships in data.
- Speed: Where traditional analytics might involve downloading and analyzing spreadsheets to answer a single question, AI analytics automates these processes – and many others.
- Scale: AI analytics can ingest large amounts of data from multiple data sources to find analytical insights that traditional approaches may miss. When combined with automation and faster processing times, organizations can scale AI analytics more efficiently than traditional analytics.
- Complexity: AI analytics can answer ambiguous questions. For example, a marketing team may use traditional analytics to segment customers by known characteristics, such as age or location. But they can use AI analytics to find segments based on undefined shared traits or interests, and the results could include segments that they wouldn’t have thought to create on their own.
The insights from data analytics might be incorporated into a business intelligence platform. Traditionally, data analysts would upload reports or update a dashboard that business leaders could use to see the results and make educated decisions. Modern business intelligence and analytics solutions allow non-technical business leaders to analyze data on their own.
With AI analytics running in the background, business leaders can quickly and easily create their own reports and test hypotheses. The AI-powered tools may even be able to learn from users’ interactions to make the results more relevant and helpful over time.
WATCH: See how organizations are using business intelligence to unlock better lending decisions with expert insights and a live demo.
Using AI analytics to improve underwriting
From global retailers managing supply chains to doctors making life-changing diagnoses, many industries are turning to AI analytics to make better data-driven decisions. Within financial services, there are significant opportunities throughout customer lifecycles.
For example, some lenders use machine learning (ML), a subset of AI, to help create credit risk models that estimate the likelihood that a borrower will miss a payment in the future. Credit risk models aren’t new — lenders have used models and credit scores for decades. However, ML-driven models have been able to outperform traditional credit risk models by up to 15 percent.1
In part, this is because the machine learning models might use traditional credit data and alternative credit data* (or expanded FCRA-regulated data), including information from alternative financial services and buy now pay later loans. They can also analyze the vast amounts of data to uncover predictive attributes that logistic regression (a more traditional approach) models might miss.
The resulting ML models can score more consumers than traditional models and do so more accurately. Lenders that use these AI-driven models may be able to expand their lending universe and increase automation in their underwriting process without taking on additional risk.
However, lenders may need to use a supervised learning approach to create explainable models for credit underwriting to comply with regulations and ensure fair lending practices.
Read: The Explainability: ML and AI in credit decisioning report explores why ML models will become the norm, why explainability is important and how to use machine learning.
Experian helps clients use AI analytics
Although AI analytics can lead to more productive and efficient analytics operations over time, the required upfront cost or expertise may be prohibitive for some organizations. But there are simple solutions.
Built with advanced analytics, our Lift Premium™ scoring model uses traditional and alternative credit data to score more consumers than conventional scoring models. It can help organizations increase approvals among thin-file and credit-invisible consumers, and more accurately score thick-file consumers.2
Experian can also help you create, test, deploy and monitor AI models and decisioning strategies in a collaborative environment. The models can be trained on Experian’s vast data sources and your internal data to create a custom solution that improves your underwriting accuracy and capabilities.
Learn more about machine learning and AI analytics.
* When we refer to “Alternative Credit Data,” this refers to the use of alternative data and its appropriate use in consumer credit lending decisions as regulated by the Fair Credit Reporting Act (FCRA). Hence, the term “Expanded FCRA Data” may also apply in this instance and both can be used interchangeably.
1. Experian (2020). Machine Learning Decisions in Milliseconds
2. Experian (2022). Lift PremiumTM product sheet