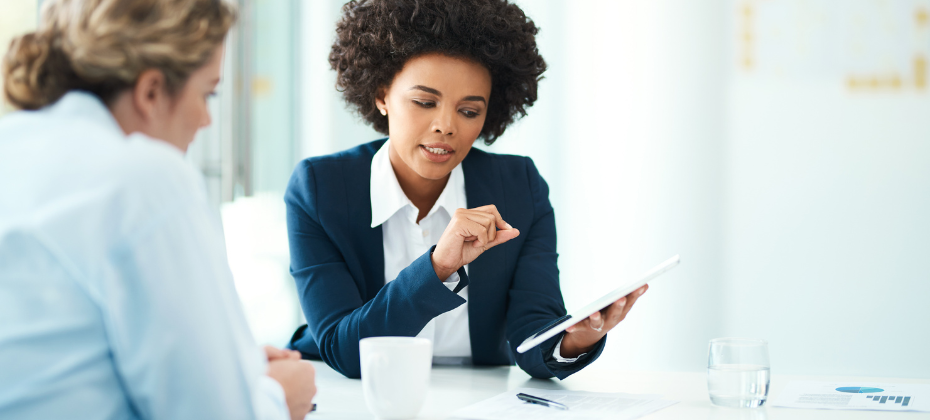
In today’s complex business landscape, data-based decision-making has become the norm, with advanced technologies and analytics tools facilitating faster and more accurate modeling and predictions. However, with the increased reliance on models, the risk of errors has also increased, making it crucial for organizations to have a comprehensive model risk management framework. In this blog post, we will dive deeper into model risk management, its importance for organizations, and the key elements of a model risk management framework.
What is model risk?
First, let’s define what we mean by model risk. Many institutions use models to forecast and predict the future performance of investments, portfolios or consumers’ creditworthiness. Model risk can happen when the results produced by these models are inaccurate or not fit for the intended purpose. This risk arises due to several factors, like data limitations, model assumptions and inherent complexities in the underlying modeled processes. For example, in the credit industry, an inaccurately calibrated credit risk modelmay incorrectly assess a borrower’s default risk, resulting in erroneous credit decisions and impacting overall portfolio performance.
What is a risk management model and why is it important?
A risk management model, or model risk management, refers to a systematic approach to manage the potential risks associated with the use of models and, more specifically, quantitative models built on data. Since models are based on a wide range of assumptions and predictions, it’s essential to recognize the possibility of errors and acknowledge its impact on business decisions. The goal of model risk management is to provide a well-defined and structured approach to identifying, assessing, and mitigating risks associated with model use.
The importance of model risk management for institutions that leverage quantitative risk models in their decisioning strategies cannot be overstated. Without proper risk management models, businesses are vulnerable to significant consequences, such as financial losses, regulatory enforcement actions and reputational damage.
Model risk management: essential elements
The foundation of model risk management includes standards and processes for model development, validation, implementation and ongoing monitoring. This includes:
- Policies and procedures that provide a clear framework for model use and the associated risks.
- Model inventory and management that captures all models used in an organization.
- Model development and implementation that documents the policies for developing and implementing models, defining critical steps and role descriptions.
- Validation and ongoing monitoring to ensure the models meet their stated objectives and to detect drift.
In addition to these essential elements, a model risk management framework must integrate an ongoing system of transparency and communication to ensure that each stakeholder in model risk governance is aware of the policies, processes and decisions that support model use. Active engagement with modelers, validators, business stakeholders, and audit functions, among other stakeholders, is essential and should be included in the process.
How we can help
Experian® provides solutions and risk mitigation tools to help organizations of all sizes establish a solid model risk management framework to meet regulatory and model risk governance requirements, improve overall model performance and identify and mitigate potential risk. We provide services for back testing, benchmarking, sensitivity analysis and stress testing. In addition, our experts can review your organization’s currentmodel risk management practices, conduct a gap analysisand assist with audit preparations.
*This article includes content created by an AI language model and is intended to provide general information.