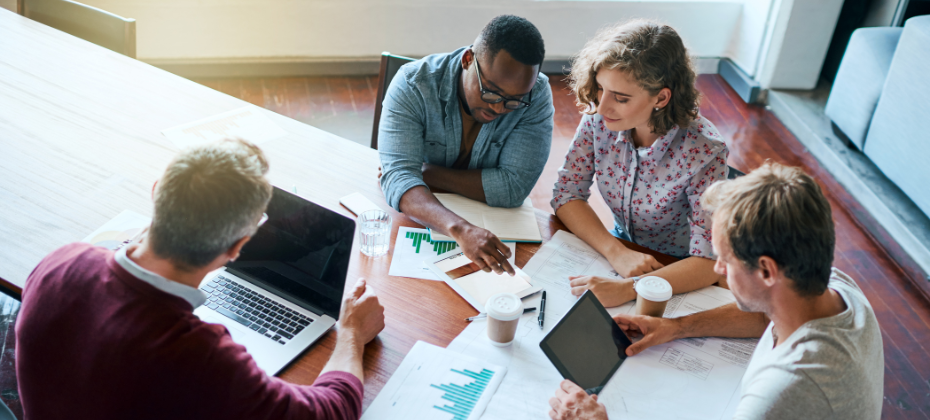
From science fiction-worthy image generators to automated underwriting, artificial intelligence (AI), big data sets and advances in computing power are transforming how we play and work. While the focus in the lending space has often been on improving the AI models that analyze data, the data that feeds into the models is just as important. Enter: data-centric AI.
What is a data-centric AI?
Dr. Andrew Ng, a leader in the AI field, advocates for data-centric AI and is often credited with coining the term. According to Dr. Ng, data-centric AI is, ‘the discipline of systematically engineering the data used to build an AI system.’1
To break down the definition, think of AI systems as a combination of code and data. The code is the model or algorithm that analyzes data to produce a result. The data is the information you use to train the model or later feed into the model to request a result.
Traditional approaches to AI focus on the code — the models. Multiple organizations download and use the same data sets to create and improve models. But today, continued focus on model development may offer a limited return in certain industries and use cases.
A data-centric AI approach focuses on developing tools and practices that improve the data.
You may still need to pay attention to model development but no longer treat the data as constant. Instead, you try to improve a model’s performance by increasing data quality. This can be achieved in different ways, such as using more consistent labeling, removing noisy data and collecting additional data.2
Data-centric AI isn’t just about improving data quality when you build a model — it’s also part of the ongoing iterative process. The data-focused approach should continue during post-deployment model monitoring and maintenance.
Data-centric AI in lending
Organizations in multiple industries are exploring how a data-centric approach can help them improve model performance, fairness and business outcomes. For example, lenders that take a data-centric approach to underwriting may be able to expand their lending universe, drive growth and fulfill financial inclusion goals without taking on additional risk.
Conventional credit scoring models have been trained on consumer credit bureau data for decades. New versions of these models might offer increased performance because they incorporate changes in the economic landscape, consumer behavior and advances in analytics. And some new models are built with a more data-centric approach that considers additional data points from the existing data sets — such as trended data — to score consumers more accurately. However, they still solely rely on credit bureau data.
Explainability and transparency are essential components of responsible AI and machine learning (a type of AI) in underwriting. Organizations need to be able to explain how their models come to decisions and ensure they are behaving as expected.
Model developers and lenders that use AI to build credit risk models can incorporate new high-quality data to supplement existing data sets. Alternative credit data can include information from alternative financial services, public records, consumer-permissioned data, and buy now, pay later (BNPL) data that lenders can use in compliance with the Fair Credit Reporting Act (FCRA).*
The resulting AI-driven models may more accurately predict credit risk — decreasing lenders’ losses. The models can also use alternative credit data to score consumers that conventional models can’t score.
Infographic: From initial strategy to results — with stops at verification, decisioning and approval — see how customers travel across an Automated Loan Underwriting Journey.
Business benefit of using data-centric AI models
Financial services organizations can benefit from using a data-centric AI approach to create models across the customer lifecycle. That may be why about 70 percent of businesses frequently discuss using advanced analytics and AI within underwriting and collections.3
Many have gone a step further and implemented AI. Underwriting is one of the main applications for machine learning models today, and lenders are using machine learning to:4
- More accurately assess credit risk models.
- Decrease model development, deployment and recalibration timelines.
- Incorporate more alternative credit data into credit decisioning.
AI analytics solutions may also increase customer lifetime value by helping lenders manage credit lines, increase retention, cross-sell products and improve collection efforts. Additionally, data-centric AI can assist with fraud detection and prevention.
Case study: Learn how Atlas Credit, a small-dollar lender, used a machine learning model and loan automation to nearly doubled its loan approval rates while decreasing its credit risk losses.
How Experian helps clients leverage data-centric AI for better business outcomes
During a presentation in 2021, Dr. Ng used the 80-20 rule and cooking as an analogy to explain why the shift to data-centric AI makes sense.5 You might be able to make an okay meal with old or low-quality ingredients. However, if you source and prepare high-quality ingredients, you’re already 80% of the way toward making a great meal.
Your data is the primary ingredient for your model — do you want to use old and low-quality data?
Experian has provided organizations with high-quality consumer and business credit solutions for decades, and our industry-leading data sources, models and analytics allow you to build models and make confident decisions.
If you need a sous-chef, Experian offers services and has data professionals who can help you create AI-powered predictive analytics models using bureau data, alternative data and your in-house data.
Learn more about our AI analytics solutions and how you can get started today.