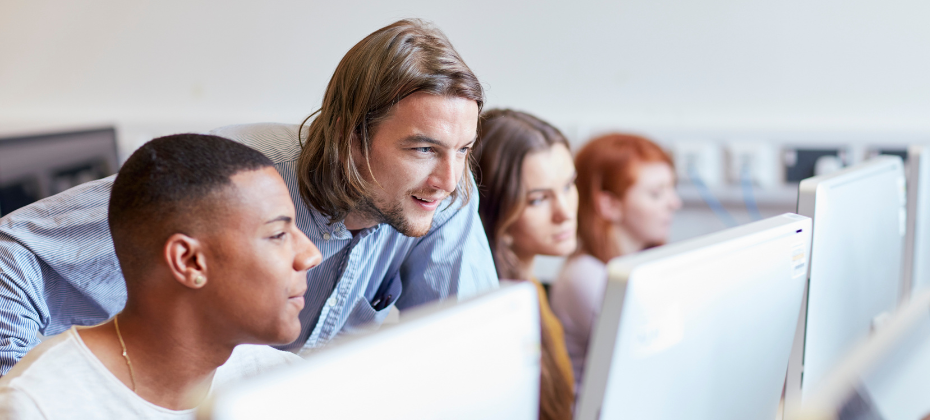
This article was updated on February 6, 2024.
Lenders looking to gain a competitive edge need to improve their credit underwriting process in the coming years. The most obvious developments are the advances in artificial intelligence (AI) — machine learning in particular — the increased available computing capacity, and access to vast amounts of data. But when it comes to credit underwriting models, those are tools you can use to reach your goals, not a strategy for success.
The evolution of credit underwriting
Credit underwriters have had the same goal for millennia — assess the creditworthiness of a borrower to determine whether to offer them a loan. But the process has changed immensely, and the pace of change has recently increased.
Fewer than 50 years ago, an underwriter might consider an applicant’s income, occupation, marital status, and sex to make a decision. The Equal Credit Opportunity Act didn’t pass until 1974. And it wasn’t expanded to prohibit lending discrimination based on other factors, such as color, age, and national origin, until two years later.
Regulatory changes can have an immediate and immense impact on credit underwriting, but there were also slower changes developing. As credit bureaus centralized and computers became more readily available, credit decisioning systems offered new insights. The systems could segment groups and help lenders make more complex and profitable decisions at scale, such as setting risk-appropriate credit limits and terms.
INFOGRAPHIC: Data-driven decisioning journey map
With access to more data and computing power, lenders get a more complete picture of applicants and their current customers. Technological advances also lead to automated decisions, which can improve lenders’ workflows and customer satisfaction. In the late 2000s, fintech lenders entered the scene and disrupted the ecosystem with a completely online underwriting and funding process.
More recently, AI and machine learning started as buzzwords, but quickly became business necessities. In fact, 66% of businesses believe advanced analytics, including machine learning and artificial intelligence, are going to rapidly change the way they do business.1
The latest explainable machine learning models can increase automation and efficiency while outperforming traditional modeling approaches. Access to increased computing power is, once again, helping power this shift.2 But it’s also only possible because of the lenders access to alternative credit data.*
WATCH: Why Advanced Analytics is Now Available for All
Future-proofing your credit underwriting strategy
Today’s leading lenders use innovative technology and comprehensive data to improve their credit decisioning — including fraud detection, underwriting, account management, and collections. To avoid getting left behind, you need to consider how you can incorporate new tools and processes into your strategy.
- Get comfortable with machine learning models
Although machine learning models have repeatedly shown they can offer performance improvements, lenders may hesitate to adopt them if they can’t explain how the models work. It’s smart to be cautious as so-called “black box” models generally don’t pass regulatory muster — even if they can offer a greater lift. But there is a middle ground, and credit modelers use machine learning techniques to develop more effective models that are fully explainable.
READ MORE: Explainability: ML and AI in credit decisioning
- Explore new data sources
Machine learning models are great at recognizing patterns, but you need to train them on large data sets if you want to unlock their full potential. Lenders’ internal data can be important, especially if they’re developing custom models. But lenders should also try leveraging various types of alternative credit data to train models and more accurately assess an applicant’s creditworthiness. This can include data from public records, rental payments, alternative financial services, and consumer-permissioned data.
READ MORE: 2023 State of Alternative Credit Data Report
- Focus on financial inclusion
Using new data sources can also help you more accurately understand the risk of an applicant who isn’t scorable with traditional models. For example, Lift Premium™ uses machine learning and a combination of traditional consumer bureau credit data and alternative credit data to score 96 percent of U.S. consumers — 15 percent more than conventional scores.3 As a result, lenders can expand their lending universe and offer right-sized terms to people and groups who might otherwise be overlooked.
- Use AI to fuel automation
Artificial intelligence can accelerate automation throughout the credit life cycle. Machine learning models do this within underwriting by more precisely estimating the creditworthiness of applicants. The more accurate a model is, the better it will be at identifying applicants who lenders want to approve or deny.
- Consider your decisioning strategy
Although a machine learning model might offer more precise insight, lenders still need to set their decisioning strategy and business rules, including the cutoff points. Credit decisioning software can help lenders implement these decisions with speed, accuracy, and scalability.
CASE STUDY: Experian partnered with OneAZ Credit Union to upgrade to an advanced credit decisioning platform and automate its underwriting strategy. The credit union increased load funding rates by 26 percent within one month and reduced manual reviews by 25 percent.
- Use underwriting as a component of strategic optimization
Advanced analytics allow companies to move away from simpler rule-based decisions and toward strategies that take the business’s overall goals into account. For example, lenders may be able to optimize decisions that involve competing goals — such as targets for volume and bad debt — to help the business reach its goals.
- Test and benchmark
Underwriting is an iterative process. Lenders can use machine learning techniques to build and test challenger models and see how well they perform. You can also compare the results to industry benchmarks to see if there’s likely room for more improvement.
Why lenders choose Experian
Lenders have used Experian’s consumer and business credit data to underwrite loans for decades, but Experian is also a leader in advanced analytics. As lenders try to figure out how they’ll approach underwriting in the coming years, they can partner with Experian’s data scientists, who understand how to develop and deploy the latest types of compliant and explainable credit underwriting models.
Experian also offers credit underwriting software and cloud-based and integrated decisioning platforms, along with modular solutions, such as access to alternative credit data, predictive attributes and scores. And lenders can explore collaborative approaches to developing ML-aided models that incorporate internal and third-party data.
If you’re not sure where to start, a business review can help you identify a few quick wins and create a road map for future improvements.
Explore our credit decisioning solutions.
* When we refer to “Alternative Credit Data,” this refers to the use of alternative data and its appropriate use in consumer credit lending decisions as regulated by the Fair Credit Reporting Act (FCRA). Hence, the term “Expanded FCRA Data” may also apply in this instance and both can be used interchangeably.
1Experian (2022). Explainability: ML and AI in credit decisioning
2Experian (2022). Webinar: Driving Growth During Economic Uncertainty with AI/ML Strategies
3Experian (2022). Lift Premium