Due to Covid-19 , the focus on analytics and artificial intelligence (AI) has significantly increased. However, while companies have made significant investments in AI, many are struggling to show a tangible impact in return.
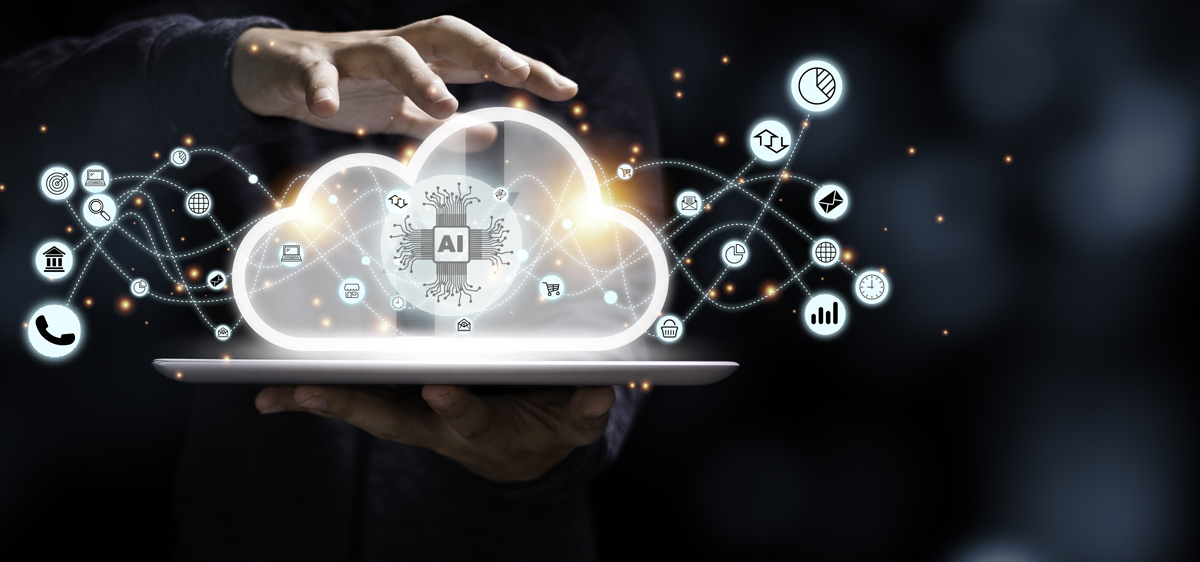
One executive commented, “We have data science teams and a data lab where advance techniques like neural networks, GANs, etc. are successfully being used. However, less than 10% of our actual operational decisions and products are powered by AI and machine learning (ML). I would like us to be driving greater measurable impact and Covid-19 is exposing some of our execution gaps.” And, he’s not alone.
Despite the investment, the true impact is elusive, and many businesses are not getting the desired effect from their efforts. Achieving the results needed to justify continuous investment will take a holistic approach. So, what can companies do to achieve this impact?
The four pillars of holistic AI: performance, scaling, adoption and trust
Achieving impact from AI requires taking a more holistic approach across four pillars — beyond just the delight of the data scientist producing a better performing model.
1. AI performance — outperforming the status quo and quantifying the impact
This pillar is where most data scientists and companies tend to focus first, for example using modern AI techniques to create an underwriting model that performs better than traditional models. The so-called ‘data science moment of truth,’ where the data scientist declares that he has built a model which outperforms the status quo by 10%.
However, it’s important to note model performance alone is not sufficient. We should look beyond the model to understand business performance. What quantifiable business impact does the 10% improvement deliver? How many more credit approvals? How much lower will the charge-off rate be? This reasoning provides the important business context around what the incremental performance means.
2. AI scaling — having the right technical infrastructure to operate models at scale
This area is often ignored. The risk with data science teams is they can see their job as being completed with creating a better performing model. However, that’s just the beginning. The next important step is to operationally deploy the model and setup the operational infrastructure around it to make decisions at scale.
If it is an underwriting model, is it deployed in the right decisioning systems? Does it have the right business rules around it? Will it be sufficiently responsive for real-time decision making, or will users have to wait? Will there be alerts and monitoring to ensure that the model doesn’t degrade? Are there clearly defined, transparent and explainable business strategies, and technology infrastructure and governance to ensure all stakeholders are aware? Is the regulatory governance around this model in place? Does the complexity in the model allow it to scale?
Too often we see data scientists and data labs create great models that can’t scale and are impractical in an operating environment. One banking executive shared how her team had developed 5 machine learning models with better performance, but were in ‘cold storage’ verse in use, because they didn’t have the ability to scale and operationally deploy them effectively.
3. AI adoption — ensuring you have the right decisioning framework to help translate business decisions to business impact
With better performing predictive models and the right technology, we now need to present the information in a way that is ‘human-consumable’ and ‘human-friendly.’ At one bank, we found they built a customer churn ML model for their front lines, but no one was using it. Why? They didn’t have the contextual information needed to talk to the customer — and the sales force didn’t have faith in it — so didn’t adopt it.
Subsequently, they built a model with a simpler methodology and more information available at their fingertips — where decisions could be made. This was immediately adopted. This pillar is where the importance of decisioning tools is highlighted. The workflow and contextual information to allow a decision to be orchestrated and made is critical in driving AI adoption.
4. AI trust – having governance, guardrails and the appropriate explainability mechanisms in place to ensure models are compliant, fair and unbiased
This final pillar is probably the most important for the future of AI — getting humans to trust it. In recent times we have seen numerous examples like the Apple Card, where the underlying principles and models have been called into question.
For scalable AI impact, we need an entire ecosystem of people who can trust AI. To achieve this effect, you need to consistently apply the right principles over time. You also need the right decisions to be explained — like adverse action calls. Explainability capabilities help manage communication and understanding of advanced analytics, contributing to established AI trust. And, when fairness and bias issues come up, you need to provide good answers as to why decisions were made.
AI is poised to fundamentally change the way we do business, and studies show that $3 to 5 trillion in global value annually, up to $15 trillion by 2030, is likely to be created. We believe the four pillars highlighted above will be key to accelerating the journey to driving positive results and capturing this value. At Experian, we are making investments to drive impact for our clients by delivering against these four pillars.